Using an RTL-SDR, RF Fingerprinting and Deep Learning to Authenticate RF Devices
Every device that transmits radio waves has a unique and identifiable RF fingerprint which occurs due to the very slightly variations in the hardware manufacturing process. This means that devices using identical transmitters of the same make and model can still be differentiated from one another.
Nihal Pasham has been using this knowledge as a way to securely identify IoT sensors and other RF devices like car keyfobs. The idea is that these unique RF fingerprints are immune to authentication spoofing which could be used to create a fake transmitter with fake data. He suggests that RF fingerprinting could be used as an additional authentication check for low cost IoT devices with only basic security.
In order to recognize the minute differences in the RF fingerprints of different devices Nihal notes that a good pattern detection algorithm is required, and that a deep learning neural network fits the bill. Using neural network software Tensorflow, and an RTL-SDR for signal acquisition, he was able to train a proof of concept neural model that was able to classify two test transmitters with 97% accuracy.
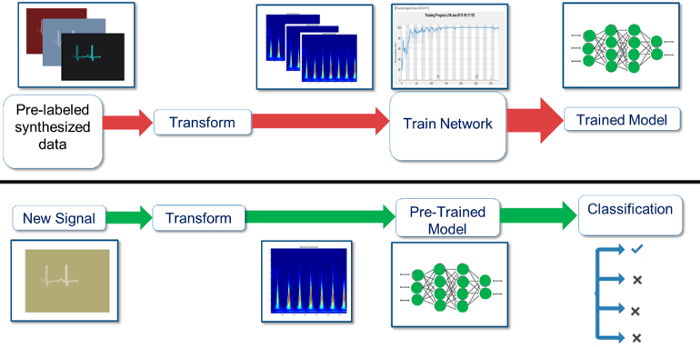
In the past we've seen similar experiments by Oona Räisänen who used an RTL-SDR to fingerprint several hand held radios heard on the air via small variances in the power and frequencies of each radio's CTCSS tone. Using simple clustering techniques she was able to determine exactly who was transmitting based upon the unique CTCSS.
In a somewhat similar fashion, Disney Research has also been working on a RF fingerprinting technique that uses an RTL-SDR based wrist watch to identify what particular electronic devices the wearer is touching.